All in on AI, Understanding AI Bias & Fairness
As AI usage begins to touch every part of our lives, it is more important than ever to ensure it is developed in a way that is fair and responsible for all people, regardless of their background.
Fairness is the idea that all human beings are of equal moral status and should be free from discriminatory harm.*The goal of fairness in AI is to design, develop, deploy, and use AI Systems in ways that do not lead to discriminatory harm.
Discrimination risks are neither new nor unique to AI, and like other technologies, it is not possible to achieve zero risk of bias in an AI System.* However, at Sanofi, we strive to ensure our AI Systems are designed to reasonably minimize discriminatory outcomes wherever possible.
The First Step in Mitigating AI Bias Is Understanding How Biases Are Introduced Into AI Systems
When considering bias, typically we consider two kinds: conscious (known) and unconscious (unknown). There are many areas where biases can be introduced throughout the AI System lifecycle:
From Data
The effectiveness of AI/ML Systems relies on the quality of their training data. Although data is often considered 'ground truth,' it remains an abstraction of our complex world. The production, construction and interpretation of data reflects our world’s ever-changing social norms and practices, power relations, political, legal, and economic structures, and human intentions.*
A common issue leading to biased or unfair outcomes is unrepresentative data – concerning either the populations involved or the phenomena that is being modelled. Throughout history, marginalized individuals have frequently been inadequately represented in datasets due to their limited access to standard healthcare within conventional health systems. Consequently, routine data collection regarding their health status and activities is often lacking. This could lead to a poorer analysis of these patient populations from AI Systems.*
In the life sciences industry, electronic health records, genome databases and biobanks often under-sample individuals with irregular or limited access to healthcare systems, such as minority ethnicities, immigrants, and socioeconomically disadvantaged groups.*
And, even when datasets are representative, they may still reflect historical and existing biases from the world.* Solving the lack of representation in datasets is a difficult task and must be addressed from both technical and non-technical perspectives. From a non-technical perspective, we are addressing one of the root causes for the lack of representativeness of data– often marginalized communities distrust the healthcare system. At Sanofi, A Million Conversations is a global initiative to rebuild trust in healthcare with the underrepresented, specifically Black, ethnic minority groups, women, people with disabilities and LGBTQ+ communities.
From Humans
All humans are influenced by their life experiences and their preferences. AI developers may add their own conscious or unconscious biases when making decisions about how to build an AI model. For example, indicators like income or vocabulary might be used by the algorithm to discriminate unintentionally against people of a certain race or gender.*
At Sanofi, AI Systems must undergo a risk assessment undergo a risk assessment, and potentially more reviews by the Responsible AI Governance Body. If an AI System is assessed to potentially lead to discriminatory outcomes, a risk mitigation plan is put into place to ensure that it meets our corporate principles for Responsible AI and ethical standards.
Unlike conscious bias, unconscious biases are difficult to capture at the start (design phase) of AI product development, but they might be discovered once the AI system are put into practice (operational phase). Knowing this, we intend to tackle this by monitoring AI systems to remain vigilant of risks, capture any unknown biases, and to put a mitigation plan into action to maintain compliance with strict standards.
How Our Teams Are Striving to Mitigate Bias in AI Systems
At Sanofi, we are developing AI models for the early diagnosis of Gaucher disease, a rare inherited genetic disorder. The intent is to allow for earlier disease detection and prompt referral to HCPs for appropriate evaluation, diagnosis, and management. Developers are taking a fairness-aware design approach – by disclosing dataset limitations, correcting bias wherever possible and detecting AI limitations through real-world testing.
Awareness and Transparency of Dataset Limitations
The journey for building an AI model that reasonably minimizes bias is a continuous improvement process; our AI developer team is exploring many different methods to minimize bias.
At Sanofi, we know that fairness, diversity, and equity in AI development is key to a future where health care is truly inclusive and accessible to all. Technology does not work unless it works for everyone. We are navigating the future of AI responsibly.
All in on AI Series
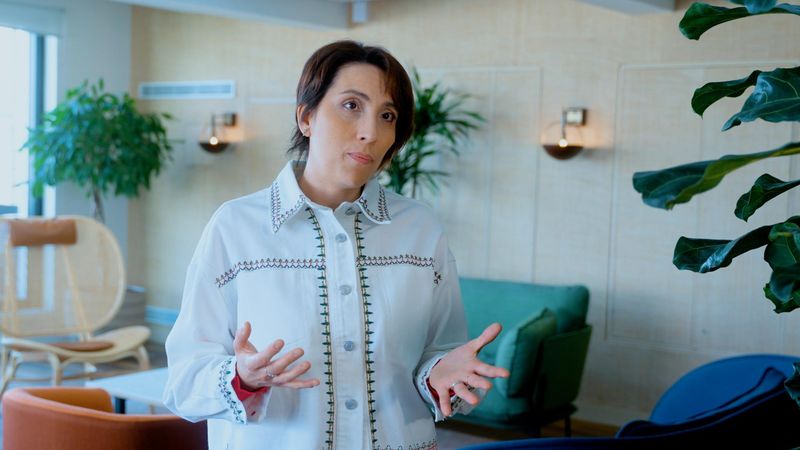
All in on AI, Accountable to Outcomes
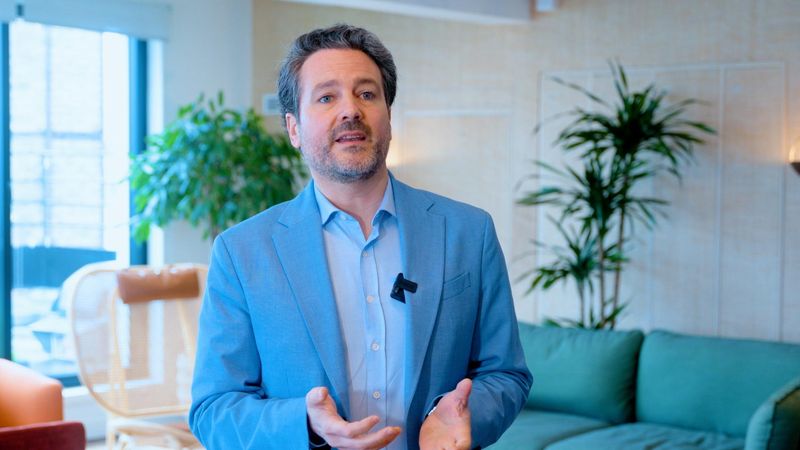
All in on AI, Environmentally Responsible
References
-
Towards a Standard for Identifying and Managing Bias in Artificial Intelligence (nist.gov)
-
Towards a Standard for Identifying and Managing Bias in Artificial Intelligence (nist.gov)
-
Towards a Standard for Identifying and Managing Bias in Artificial Intelligence (nist.gov)
-
Towards a Standard for Identifying and Managing Bias in Artificial Intelligence (nist.gov)